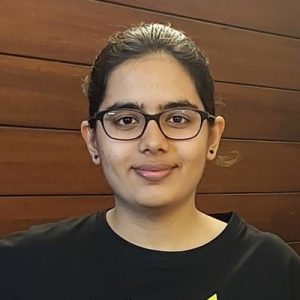
I am a final year student at the Birla Institute of Technology and Science Pilani, pursuing Mechanical Engineering.
I am extremely grateful for the opportunity to pursue my research internship under the guidance of Professor Debasish Ghose, Senior Professor at the Robert Bosch Centre for Cyber-Physical Systems and Department of Aerospace Engineering, IISc.
I have always been enthusiastic and passionate about the field of robotics and automation. Throughout my undergrad, I have developed a deep fascination for autonomous vehicles and unmanned aerial vehicles (UAVs), especially the complex interactions and systems that enable them to function synchronously.
About my research
My current research at IISc is based on creating automated drone corridors, using AI/ML tools, in a 5G network, ensuring continuous and stable communication between UAVs and 5G base stations throughout the entire flight path. By combining pathfinding algorithms, signal modelling, and machine learning, I aim to ensure stable connectivity and obstacle avoidance.
I am incorporating neural networks to predict 5G signal intensity at points where the required information is not available. The project aims to leverage AI/ML tools to produce multiple conflict-free drone corridors with sufficiently high tracking accuracies and safety margins. This can be achieved by training the AI/ML tool on the 5G network node locations and available terrain data.
By integrating the dynamics of UAV motion with 5G network architecture, I am gaining hands-on experience in an area that combines both my technical skills and research interests.
With the wonderful start and the strong foundation that I have got at IISc, I would like to continue my research in this field and build further upon it, identify the real-world challenges for which my research can be applied, to make the systems more robust and agile.