Tarun Kalluri, PhD student, Visual Computing Group, UC San Diego
– 26 February 2024
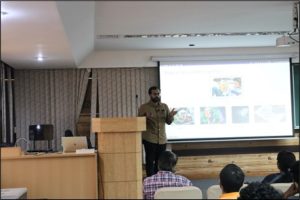
Talk summary: While recent progress significantly advances the state-of-the-art in computer vision across several tasks, the poor ability of these models to generalise to domains and categories under-represented in the training set remains a problem, posing a direct challenge to fair and inclusive computer vision. In his talk, Tarun Kalluri talked about his recent efforts towards improving generalisability and robustness in computer vision using domain adaptation. First, he spoke about work on scaling domain adaptation to large scale datasets using metric learning. Next, he introduced a new dataset effort called GeoNet aimed at benchmarking and developing novel algorithms towards geographical robustness in various vision tasks. Finally, he talked about the latest research studying the role of language supervision to improve adaptation of visual models to new domains.
Speaker bio: Tarun Kalluri is a fifth year PhD student at UC San Diego in the Visual Computing Group. Prior to that, he graduated with a bachelors from Indian Institute of Technology, Guwahati and worked as a data scientist in Oracle. His research interests lie in label and data efficient learning from images and videos, domain adaptation and improving fairness in AI. He is a recipient of IPE PhD fellowship.
[Talk organised in collaboration with the Department of Computational and Data Sciences]